Enter The Next Wave of Technological Innovation in Medical Research & Healthcare
Artificial intelligence (AI) plays an important role in our everyday lives. In recent years, it’s become the centre of attention in various business sectors from communications to research. When it comes to science, AI has significantly impacted various fields including, among others, image analysis or natural language processing and is spreading out to different areas beyond informatics including life sciences.
AI, however, is not a new discipline. The term was coined decades ago in 1956. Since then, AI evolved as a part of computer science and went through numerous cycles of increasing and decreasing popularity.
A Short Recap on AI and Why It’s a Big Deal
An oversimplified explanation of AI is where computer algorithms work together to approximate conclusions without direct human input. By constituting machine learning and deep learning (sub-disciplines of AI), computers can ‘learn’ without being explicitly programmed every step of the way.
The basic principle dictates that AI is machine intelligence that’s able to provide the best outcome when given a problem. In life sciences, one of the biggest problems researchers (and healthcare providers) face is analyzing oceans of data.
AI can be applied via four major approaches in life sciences:
- Machine learning – Processes that analyze input data and then repeatedly optimize their methods based on generated outputs
- Deep learning – A machine-learning-based approach that utilizes a logic structure akin to biological neural networks
- Natural language processing – A refined automatic speech recognition system that can interact with people through dialogue, going beyond simple reactions to well-stylized user requests
- Robotics and the internet of things (IoT) – Integration of devices to collect, combine and share different types of information
AI is enabling researchers to develop cures faster, doctors to deliver more personalized and effective care, and healthcare companies to reduce costs while increasing access to care.
According to L.E.K. Consulting, all the largest 10 pharmaceutical companies (by revenue) have either partnered with or acquired AI companies to leverage the sheer potential the technology presents.
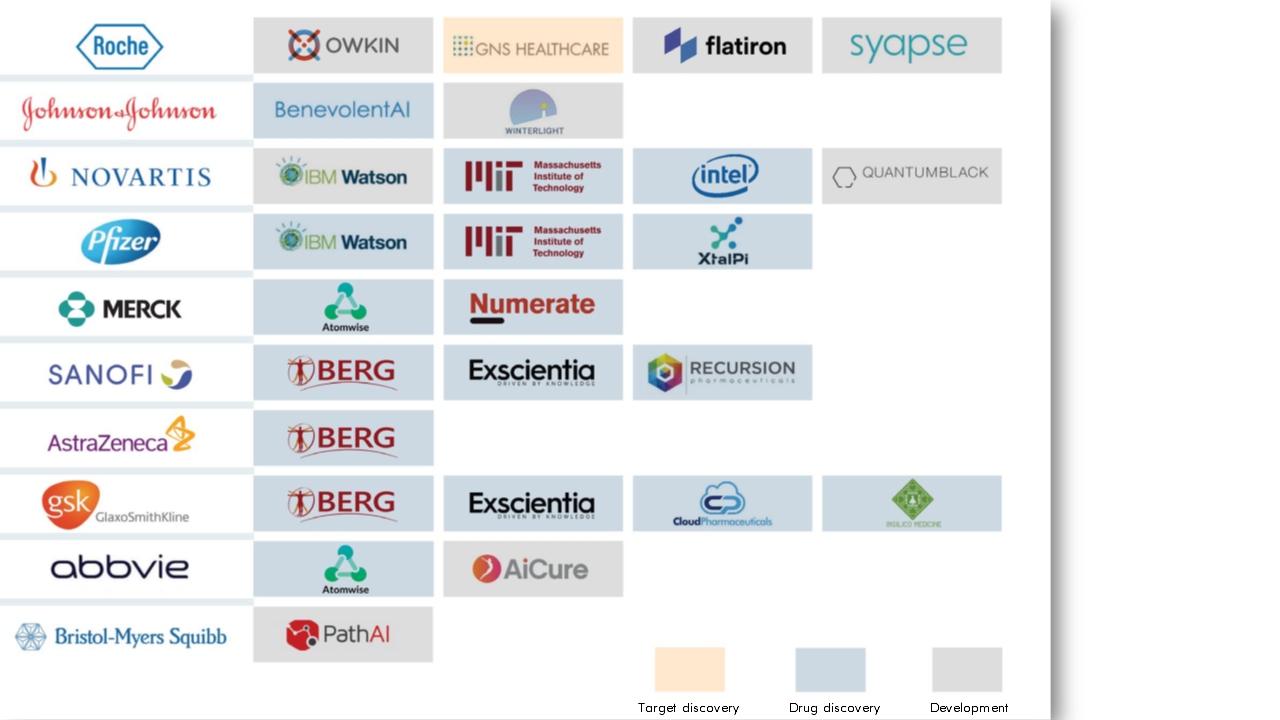
Congenica: AI-Powered Genomic Analysis
Genomics is an interdisciplinary field of biology focusing on the structure, function, evolution, mapping, and editing of genomes. A genome is a person’s complete set of DNA, including all of his/her genes. While genetics is the study of individual genes and their roles in inheritance, genomics aims at the collective characterization and quantification of all of a person’s genes, their interrelations and their influence on a person.
Genomic analysis for that matter involves a huge number of bases (approximately 3.2 billion) to be analyzed for a single person. Needless to say, analysis of genomic data is complex and time-consuming, and there are enormous amounts of data to manage.
The process of genomic interpretation can typically take more than 20 hours (or even days), and labs that perform such tasks are often nearing full capacity. By implementing AI, Congenica can reduce that time to under 30 minutes (in some cases to as little as 5 minutes) while increasing throughput up to 95%.
With AI, oceans of data can be fed directly to the deep learning model, where the computer would find meaningful features or relationships of a person’s genome. Congenica’s Clinical Decision Support platform can improve research outcomes with a 30% higher analytical yield than rare disease averages across all diseases, sample types and family structures.
Genomic medicine is vast and offers opportunities to improve healthcare across the breadth of medical specialities. Clinical applications include:
- Gene discovery and diagnosis of rare diseases
- Identify genetic factors contributing to common disease
- Pharmacogenetics and targeted therapy – genetic information can be used to predict how a patient will respond to a specific treatment
- Prenatal diagnosis and testing – identify genetic causes of fetal anomalies during pregnancy
- Sequencing infectious diseases
- Personalized medicine – use of genetic information to tailor healthcare intervention to a patient’s individual need
- Gene therapy
- Genome editing
InterVenn: AI-Powered Liquid Biopsy
A liquid biopsy is a form of diagnostic done on a sample of blood to look for cancer cells from a tumour that are circulating in the blood or for pieces of DNA from tumour cells that are in the bloodstream. Unlike conventional standard biopsy that is time-intensive and invasive, liquid biopsies are quick yet comprehensive and more sustainable for the patient.
Liquid biopsies can be used to:
- Help screen or identify cancer at an early stage
- Help healthcare providers plan for a course of treatment
- Determine whether a treatment is working, or whether a patient would risk a relapse
- Help doctors understand what kind of molecular changes are taking place in a tumour (via multiple samples of blood taken over time)
InterVenn is developing a set of innovative solutions that leverages the rich diversity of protein glycosylation, the process of attaching sugar molecules to a variety of biological materials such as proteins, lipids and even RNA. Glycosylation is essential for normal cell and organ functions, but undesired alterations can lead to various diseases, including cancer.
Glycans, also called polysaccharides, are carbohydrate-based polymers made by all living organisms. Glycans are one of four major classes of macromolecules present in all lifeforms, the other three being lipids, proteins, and nucleic acids.
Driven by complex biosynthetic pathways involving genetic and environmental factors, glycans are adaptive to environmental changes. Therefore, glycosylation dynamically affects protein structure and function. Aberrant glycosylation of proteins has been implicated in key stages of disease biology, including cancer as well as inflammation cascades associated with autoimmunity and ageing.
Historically, glycoproteomics has been analytically and computationally challenging to study at scale. Unlike DNA, RNA, and proteins, glycans are structurally complex and their synthesis is not template-based.
With advanced analytics and AI-powered software, InterVenn’s deep-learning platform allows high-throughput glycoproteomic analysis for both medical researchers and healthcare providers.
Conclusion
AI has significantly impacted various fields and is spreading out to different areas beyond informatics including life sciences. AI has the potential to tackle one of the biggest problems medical researchers (and healthcare providers) face: the analysis of huge amounts of data. From drug discovery and clinical research to diagnosing cancer and other rare diseases, AI has the potential to disrupt the way we view medicine, research, health, and well-being.
Sources:
- https://www.sciencedirect.com/science/article/pii/S2667318521000015
- https://www.news-medical.net/life-sciences/Artificial-Intelligence-and-Life-Science.aspx
- https://www.lek.com/sites/default/files/insights/pdf-attachments/2060-AI-in-Life-Sciences.pdf
- https://www.nature.com/subjects/genomic-analysis
- https://www.genome.gov/health/Genomics-and-Medicine
- https://www.futurelearn.com/info/courses/the-genomics-era/0/steps/4911
- https://www.congenica.com/solutions/our-platform/
- https://www.cancer.gov/publications/dictionaries/cancer-terms/def/liquid-biopsy
- https://www.biochain.com/general/a-tale-of-two-biopsies-liquid-biopsy-vs-tissue-biopsy/
- https://intervenn.com/glyco/
- https://en.wikipedia.org/wiki/Artificial_intelligence_in_healthcare
- https://en.wikipedia.org/wiki/Genomics