How AI-Based Technology can Improve the Diagnosis & Treatment of Rare Diseases
A rare disease is defined as a low-prevalence condition that affects fewer than one in 2,000 people. Given the rarity of cases, healthcare providers are often ill-equipped to diagnose and treat patients with rare diseases.
Rare diseases represent a real emerging global public health priority. So far, around 7,000 distinct rare diseases have been recognized, affecting 300 million persons globally, including 4-6% of the European population and almost 10% of the US population. From a clinical perspective, rare diseases are extremely diverse (heterogeneous) and complex, often characterized by different clinical subtypes and overlapping phenotypic manifestations.
Although most rare diseases are classified as genetic diseases (80% of rare diseases have a genetic basis), the causes remain unclear for many of them, making it difficult to identify therapeutic options. In a nutshell, rare diseases are responsible for enormous healthcare costs, mainly due to difficulties in diagnosis and their often-serious health degenerative implications over time.
The challenges to patients with a rare disease are threefold:
- They may experience the manifestations of the disease but struggle to find physicians knowledgeable about their condition (to identify and treat);
- They may suffer the consequences of the disease and go completely unrecognized; and
- They may be faced with very high treatment costs (medications and procedures).
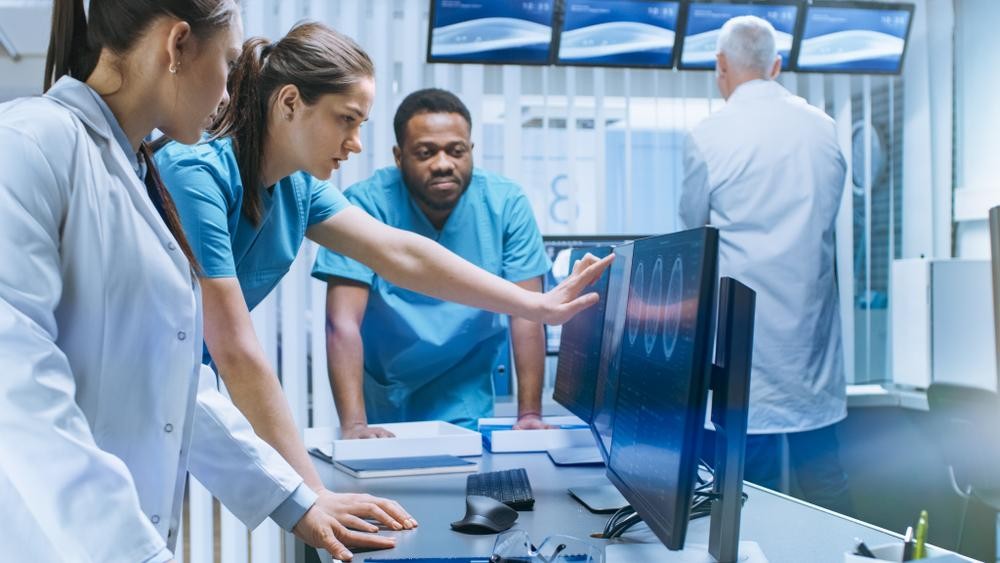
The Complexity of Cases & Scarcity of Reliable Data
The National Organization for Rare Disorders (NORD) surveyed over a thousand people about living with a rare disease. It is found that 28% of those surveyed had to wait 7 years (or more) for an accurate diagnosis. Approximately 38% of all the participants received a misdiagnosis during their journeys. In search of a diagnosis, patients endure endless testing and expenses, while missing out on years of possible treatment.
Further compounding the issue are treatment options for rare diseases. Of the more than 7,000 rare diseases described worldwide, only 5% have a treatment available.
In addition, not all the research on rare diseases gets published. While the number of published studies has increased tremendously in recent years, experts believe that’s just the tip of the iceberg.
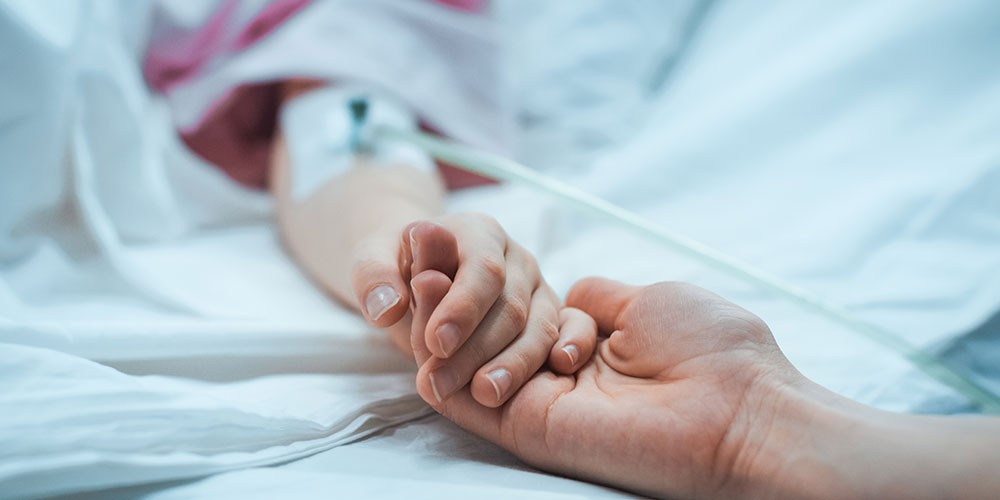
Bringing Artificial Intelligence into the Mix
A quick recap: artificial intelligence (AI) comprises computer algorithms that work together to approximate conclusions without direct human input. By constituting machine learning and deep learning (sub-disciplines of AI), computers can learn without being explicitly programmed every step of the way.
In short, AI is machine intelligence that’s able to provide the best outcome when given a problem. In life sciences, one of the biggest problems researchers (and healthcare providers) face is analyzing oceans of data, ranging from publications, numbers, and statistical trends, even images.
The amount of data healthcare systems gather is both large and diverse in format, it is difficult hard for humans to make sense of it on their own. AI can comb through data to extract information and recognize patterns.
While humans get tired, machines do not. No matter how much data is being fed, AI would still be able to process them and output results. Humans have limitations to how much they can handle and how much work they can do.
Healthcare professionals can utilize AI in a variety of ways, including:
- Diagnosing patients
- Defining disease characteristics
- Identifying potential treatments and which conditions will benefit from the treatments
- Predicting disease progression or response to treatment
- Understanding how a disease associates with other conditions
Patient privacy is a critical issue, and AI can be regulated such that analysis doesn’t include any personally identifiable information (PII) and is compliant with the Health Insurance Portability and Accountability Act of 1996 (HIPAA).
In a separate study by Nuance, a global survey revealed that 60% of people would be happy to let AI participate in their medical records. There is already support out there for a faster, more accurate system of reviewing records.
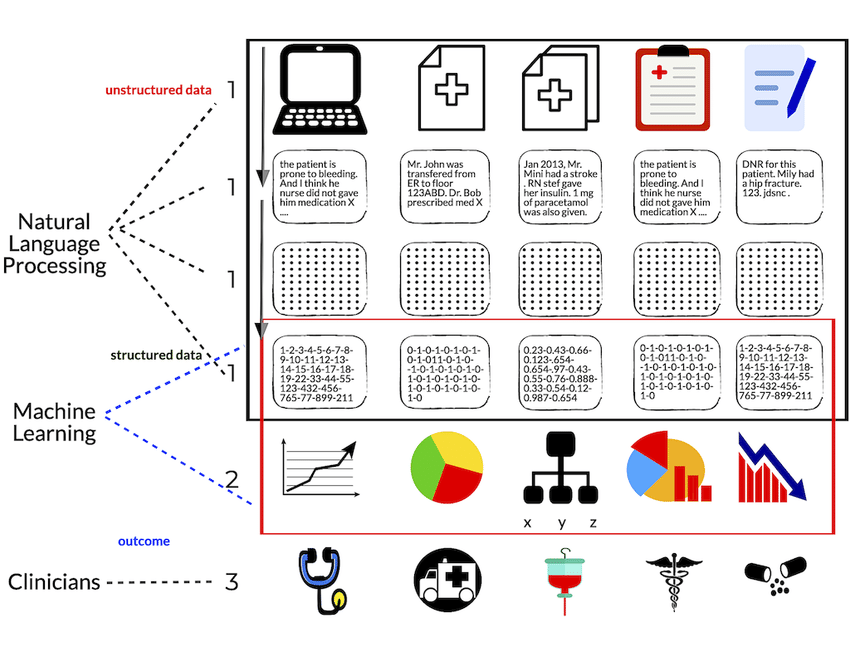
Schematic illustration of how natural language processing converts unstructured text to machine-readable structured data, which can then be analyzed by machine-learning algorithms (Choudhury & Asan, July 2020).
Zooming in: Natural Language Processing (NLP)
To reduce manual searches (that is time-consuming) and improve their findings, many organizations are turning to AI technologies such as natural language processing (NLP). To summarize, NLP is a branch of AI that helps computers understand, interpret and manipulate human language.
With NLP, organizations can quickly (and accurately) assess and streamline greater volumes of clinical data. Clinical and research institutions are adopting NLP to capture unstructured information from electronic health records (EHRs) to improve diagnosis and identification.
In the case of rare diseases, NLP offers many benefits to:
- Patients: AI minimizes the time, money, and effort it takes to get a diagnosis. An earlier diagnosis provides an opportunity for earlier treatment, which typically improves outcomes. AI can identify approved therapies and clinical trials related to rare diseases.
- Healthcare Providers: NLP tools give doctors the help they need to diagnose rare diseases. AI predictions are usually precise and reduce the element of human error in the diagnostic process.
- Payers (Health Insurance): A timely and accurate diagnosis saves payers from the costs of unnecessary tests and misdiagnosis. When patients receive treatment earlier, it can lessen the number of health concerns that may develop when untreated over time.
Case Studies of AI in Rare Diseases
The following are several success stories that show the potential of AI in combating rare diseases:
Congenica Clinical Decision Support – A platform for genomic analysis, it enables organizations to rapidly interpret next-generation sequencing data within minutes (instead of days), maximizing case throughput up to 95% and improving health outcomes. It is available as a CE Marked IVD clinical decision support platform for clinical diagnostic use for inherited genetic disorders across Europe. Organizations that use Congenica’s platform include Roche, Veritas, and the United Kingdom’s National Health Service (NHS).
Intervenn’s GLORI™ – GLORI is the world’s first glycoproteomic, liquid-biopsy laboratory-developed test (LDT). Specifically for ovarian cancer diagnosis, it has been validated using both retrospective patient samples and samples collected prospectively in InterVenn’s VOCAL clinical trial. Ovarian cancer affects about 1 in 70 women in the US, and the average age at diagnosis is 63. Worldwide about 240,000 women are diagnosed each year with ovarian cancer.
Fabric GEM AI – A study done across six genomic centers and hospitals found that more than 90% of disease-causing variants in infants were identified by this AI algorithm. The research also used Clinithink’s CLiX focus, a natural language processing (NLP) technology applied to medical notes, which are recorded in electronic medical records in the US. In contrast to letting humans read medical notes, this method provided faster results.
AI to Play Pivotal Role in the Future of Healthcare
According to ResearchAndMarkets.com, more than USD 10 billion has been invested in the AI-powered drug discovery industry in the last five years. Half of that was invested within the last two years, reflecting the increasing interest of stakeholders in AI-based tools for drug discovery.
Currently, about 210 companies claim to offer AI-based services, platforms and tools for drug discovery (including those for treating rare diseases).
According to Precedence Research, the global AI in healthcare market size is predicted to reach approximately USD 187.95 billion by 2030 and expand growth at a CAGR of 37% from 2022 to 2030.
Prophecy Market Insights predicted that rare disease treatments continue to be a trending sector for drug makers; with the projected market value to grow from USD 161.4 billion in 2020 to more than USD 547 billion by 2030.
Conclusion
As advances in technology reveal new uses for AI, its role in the medical space continues to grow. Experts are also learning how to focus AI to meet specific challenges, especially in the case of rare diseases.
Sources:
- https://www.frontiersin.org/articles/10.3389/fmed.2021.747612/full
- https://www.openaccessgovernment.org/diagnosing-rare-diseases/122418/
- https://www.sciencedirect.com/science/article/pii/S0012369218300643
- https://www.nature.com/articles/s41588-022-01037-8
- https://www.gaucherdisease.org/blog/ai-and-rare-disease-diagnosis-national-gaucher-foundation/
- https://pubmed.ncbi.nlm.nih.gov/34809862/
- https://www.researchgate.net/publication/337616881_Artificial_Intelligence_AI_in_Rare_Diseases_Is_the_Future_Brighter
- https://nationalpress.org/topic/ai-driving-breakthroughs-on-rare-diseases/
- https://medcitynews.com/2022/03/improving-the-diagnosis-and-treatment-of-rare-diseases-with-ai-based-technology/
- https://www.researchgate.net/publication/343194021_Role_of_Artificial_Intelligence_in_Patient_Safety_Outcomes_Systematic_Literature_Review
- https://www.fiercepharma.com/marketing/for-rare-disease-drugmakers-ai-and-real-world-data-can-help-find-elusive-patients
- https://www.globenewswire.com/en/news-release/2022/04/11/2420243/0/en/Artificial-Intelligence-in-Healthcare-Market-Size-to-Hit-US-187-95-Bn-By-2030.html
- https://www.sas.com/en_us/insights/analytics/what-is-natural-language-processing-nlp.html